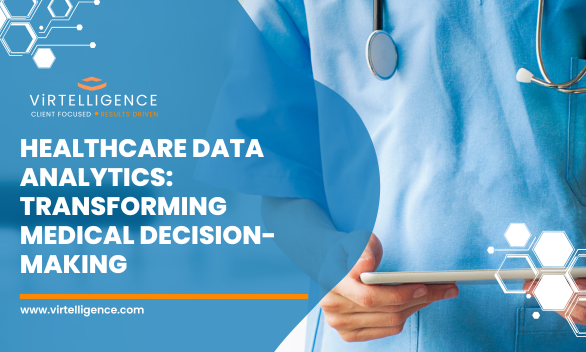
Data analytics in the current dispensation of fast-changing trends and improvements in the healthcare provision can be termed as a ‘game-changer’ in matters relating to medical decision-making and patient management.Healthcare Data Analytics uses artificial intelligence and machine learning to curate and analyze large data sets, including electronic health records and medical images.
Through these sophisticated interventions, the providers have utilized evidence in the databases to close the gaps that lead to ancillary outcomes but impact patients’ overall well-being beyond their primary diagnosis.
That is why when you step into the field of healthcare data analytics, you will see it has been applied across every field of healthcare and medical studies. This article will take you through the wanted details of the healthcare data analytical method concerning the positions described in predictive analysis, population care management, and tailored medication.
You will also learn about expanding the domain of healthcare data analytics jobs, certifications, and courses to further your career in this emerging and exciting field. Get ready to discover how healthcare data analytics is transforming patient outcomes and the future of the healthcare sector.
What is Healthcare Data Analytics Certification
Healthcare data analytics certification validates your expertise in analyzing and interpreting healthcare data. It demonstrates your ability to transform raw data into valuable insights, enabling better decision-making in healthcare organizations. The Certified Health Data Analyst (CHDA) designation, offered by AHIMA, is a prominent certification within the field of healthcare data analytics.
To qualify for the CHDA exam, you need to hold an RHIT or RHIA credential or have a bachelor’s degree or higher from an accredited institution. While not mandatory, it’s recommended to have at least three years of healthcare data experience.
The examination that is offered by the CHDA is administered in six domains, and one of them relates to data mining, manipulation, and analysis of large sets of data. You have 3.5 hours to complete the computer-based exam and a passing score of 300.
Gaining this certification demonstrates your mastery in the procurement, administration, and delivery of healthcare information. It also underlines that the candidates have good communication skills interacting with different entities – internal and external to the company.
Why CHDA Certification Important
CHDA certification not only proves that you are proficient in procurement, administration and delivery of healthcare information but also places you at the fore front of the quickly growing Healthcare data analytics Industry. This makes it easier for you to be sure that you have the knowledge and skills that are necessary in order to improve the quality of patient care, improve the efficiency in the organization.
Real-World Applications
Some of the certified health data analysts have worked afterwards at various hospitals, insurance companies and in healthcare technology companies. For instance, certified professionals have provided a good example of how experts have put into practice healthcare data analysis for the purpose of avoiding hospital readmissions, or coordinated proper use of medical personnel and drugs among others together with satisfying clients.
Healthcare Data Analytics Courses Details
Healthcare data analytics courses provide a varied number of options to develop the skills in the given progressively developing sector. Finally, Coursea has specific courses meant for health IT vendors, administrators, and medical facilitators. These courses blend social and technical environments so that you can have practical experience in health informatics.
Each of the individuals who want to know more about the utilization of artificial intelligence in healthcare can join a course from Coursera that is based on specializations and deals with the basics of artificial intelligence. You will also find information on clinical data analysis, employing machine learning, raising the security level in AI-based applications for healthcare, and more.
With the Rotman School of Management online program, you are given a framework to ask the right questions to your data analysts while developing your own analytical skills. The course will also offer lessons on how to employ data in the process of initiating cultural change in an organization.
Another popular course on the same platform deals with management of health systems and care facilities using data analysis techniques. It includes measures in data literacy in the health industry, deploying data models, developing analytical solutions, and data quality management.
Types of Healthcare Data and Their Sources
Healthcare Data Analytics can be defined as the process of using various types of data from different sources to improve patient outcomes, streamline operational processes, and provide support for making effective decisions.
Thus, it can be concluded that the value of healthcare data analytics is based on its capability to manage large amounts of information to reveal insights that may contribute to the better functionalization of the healthcare system and the preservation of people’s health.
Healthcare data analytics is like having a smart assistant that helps keep people healthier. Through collecting information from different sources, it helps doctors catch problems early. Hence, tailor treatments to individual needs, and even prevent illnesses before they start. This approach cuts down on hospital visits plus also supports better overall health, making a real difference in people’s lives.
Electronic Health Records (EHRs) and Electronic Medical Records (EMRs)
A prime data source in healthcare data analytics is Electronic Health Records (EHRs) and Electronic Medical Records (EMRs). Such records keep a detailed history of a patient’s health profile comprising diagnosis details, treatment administered, prescription details and investigations conducted among others. Through consolidating EHR and EMR data, caregivers can have a complete picture of patients’ treatment history meaning that identifying a disease, planning of treatment as well as coordination of care in multiple care settings would be easier to manage.
- EHR and EMR aid in the sharing of data and information between different healthcare providers attending to a patient, thereby reducing medical errors and duplication of tests.
- Predictive analytics using these records can identify patients at high risk for specific disease conditions or health conditions, hence enabling timely preventive measures for negative health outcomes.
Administrative Data in Healthcare Analytics
Another element that is equally important in healthcare data analytics is Administrative Data. It is obtained from patient encounters with healthcare organizations and encompasses details like admission and discharge notes, procedure codes, and charges. Administrative data can be readily extracted from an organization’s records, and healthcare data analytics can be used to process the records.
In healthcare data analytics, management can determine how efficient certain parts of their healthcare organization are. Furthermore, healthcare data analytics can assess whether the cost of their healthcare services is fair to the client and whether they are using their resources wisely.
- Administrative data is, therefore, useful in tracking the performance of the healthcare delivery system as well as the satisfaction level of the patients, thus aiding the providers to work out the best strategies for the improvement of the healthcare service delivery system.
- It offers the basic operational data regarding appointment of more personnel to hospitals and other resources such as equipment, to avoid situations where some resources are overstretched, while others are underutilized with negative impact on patient’s care. Healthcare data analytics ensures that these decisions are backed by data, leading to more efficient resource allocation and improved patient outcomes.
Healthcare data analytics not only optimizes resource allocation and operational efficiency but also enhances patient satisfaction and care quality. From the analysis of admission and discharge data, the healthcare organizations can easily discover the areas that are inefficient and which impact on the patient.
Healthcare data analytics enables healthcare organizations to monitor and improve the time spent on the delivery of care to patients. This therefore makes the healthcare system to be more focused and therefore improves the quality of care for the patients.
Claims Data: Financial Insights in Healthcare
Another source of information in healthcare data analytics is the claims data, which comprises all the outlets that patients have billed to health insurers for any type of interaction with the healthcare system.
This data is more of a record of the services offered, the expenses, and the methods used to claim reimbursement for healthcare expenses. This way, there is a better understanding of healthcare needs, financial performance of service lines, and compliance with the billing guidelines.
- Having information about claims helps to define potential items related to fraud and abuse in billing for medical services and on this basis, produce bills that would be classified as suspicious.
- It also helps evaluate costs and revenues or returns for healthcare interventions and thus helps in delivering efficacious and cost-efficient healthcare services that promote value-based care.
It is necessary to understand how all this is related to other components of healthcare analytics such as EHRs and patient feedback. When such data is integrated with clinical results and patient experience, healthcare data analytics can help providers come up with a comprehensive picture of patient management and hence better assessment of the treatment results and the general health status of the patient.
Plus, with this integrated data, and by using advanced healthcare data analytics, providers can gain insights and recognize patterns useful for making right decisions, like identifying patients who present a high risk or the best way to manage resources in order to enhance both the quality of the care and the financial results.
The Role of Patient Registries
Patient Registries that compile data on particular ailments, diseases or interventions are critical in establishing trends and assessing the impact of various health conditions. These registries are very useful in healthcare data analytics as using them, researchers and healthcare providers can monitor efficacy of treatments, identify emerging health trends in specific patient populations. and design appropriate intervention to enhance the quality of life.
- Healthcare data analytics helps Patient registries gather reliable outcome data that can be used to optimize clinical care as demonstrated by the impact on the development of clinical guidelines and improvement of patient management.
- Healthcare data analytics also plays a crucial role in chronic diseases and treatment outcomes as well as in few-year disease dynamics, so that the healthcare practitioners may make recommendations on its further management depending on the patients’ conditions.
National Health Surveys and Public Health
Healthcare data analytics relies on national health surveys, among other sources of information. Such are studies which establish the characteristics and health needs of populations in order to inform public health programmes and policies.
Healthcare data analytics and survey data points make it easier for the healthcare organizations to understand community medicine trends and evaluate the impacts of specific interventions and come up with ways of addressing health disparities.
- National health surveys show what risks communities face at large concerning their wellbeing; they also indicate preventive care requirements that ought to be met by local authorities concerned with social welfare promotion strategies.
- The data shows where resources need to go so that all people get served equally well. These findings establish two things: one being the fact that investments should target underserved regions or groups and secondly there must be even distribution of service delivery points throughout a given geographical area.
Clinical Trial Data: Evaluating Medical Treatments
Healthcare Data Analytics heavily depends on clinical trial data which comes from research studies conducted to assess the safety and effectiveness of medical treatments. This type of information is used in healthcare data analytics in determining how new drugs work under real world conditions thereby supporting the formulation of evidence-based guidelines and guiding clinical decision-making processes.
- Prior to large-scale adoption it ensures that any treatment meets safety standards besides being effective in curing diseases thus providing strong grounds for treatment choice by clinicians involved in patient care.
- Further still it enables refining protocols used during management procedures since such methods are continuously improved upon following feedback received from different quarters based on actual happenings around us when we are unwell.
In addition, specific national health surveys, including the National Health and Nutrition Examination Survey (NHANES) in the United States, have helped to define and direct public health practice. These surveys are very important data which assist in the establishment of the health status of the population and are therefore used in the formulation of policies to enhance the health of the population.
The surveys offered data on aspects like obesity and hypertension, which were used to design particular actions. Therefore, when applied in healthcare data analytics, the impacts on public health are huge, improving the outcomes and the efficiency of resource utilization. Survey data therefore needs to be transformed into effective public health policies and this is where healthcare data analytics comes in.
The Importance of Genomic Data
Genomic data is very important in healthcare data analytics. It contains information on how an organism’s genetic makeup is organized and can be used to create a treatment model specific to an individual’s genetic makeup.
- Genomic data paves the way for precision medicine which makes it possible to have remedies for almost every general illness based on one’s genes; this helps in increasing the efficiency of core healthcare services.
- It also assists in determining the hereditary tendencies of particular diseases, highlighting the possibilities of an early diagnosis and effectively planning medical treatments that can greatly enhance the given patient’s quality of life.
Ensuring Data Accuracy and Reliability
The final thing is that healthcare data analytics needs to have the correct and reliable data to ensure accuracy. This implies that information must be collected in a consistent manner, and standardized definitions and procedures must be followed.
The comparability of data is thus made feasible on account of this approach, which enables fair assessment of performance results by removing any bias or distortion, allowing for meaningful analysis across different providers and health plans.
Achieving these objectives depends on healthcare data analytics as it guarantees the accuracy and dependability of the data used, which is necessary for decision-making. Without healthcare data analytics, the ability to assess and improve healthcare outcomes would be significantly hindered.
- By using standardized dataset formats, healthcare organizations can compare their performance with industry benchmarks, identify areas for improvement, and learn from best practices.
- It guarantees that reliable and high-quality data informs decision-making in healthcare, leading to better outcomes in the market.
What is Healthcare Data Analytics Degree
A Healthcare Data Analytics degree prepares an individual with adequate knowledge to navigate the growing field of healthcare data analytics. In addition, this particular area of study aims to combine healthcare information with complex analysis tools you need to learn in order to learn how to turn raw data into useful and very effective insights to enhance patients’ care and organizations’ productivity.
As the focus has shifted to using big data and analytics to make efficient and accurate decisions in the healthcare industry, graduates with a degree in healthcare data analytics are in great demand.
Educational Pathway
Usually, it starts with acquiring a bachelor’s degree in an area that would be useful for healthcare data analytics, such as data science or health information technology. These basic programs include competencies that are core to a candidate who wants to work in healthcare data analytics.
In your undergraduate course, you will study the healthcare information systems that cover the processes of data collection, storage as well as use in various healthcare facilities.
You will also learn information management procedures in which you will specialize in organizing and preserving large volumes of health data. The information governance concept is another relevant area that focuses on the legal and proper processing of data in an organization.
- Healthcare Information Systems: Mastering the process through which data is acquired and implemented in other systems within the healthcare setting is helpful in enhancing the organization of the field and the improvement of patient care; therefore, it is crucial in healthcare data analytics.
- Data Management: Control over the management and storage of datasets is critical since it affects the quality of information in addition to its availability for analysis – so vital in the field of healthcare data analytics.
If you want to take the next step in your career journey and further your educational experience, an online master’s degree in healthcare data analytics is the next logical step.
In addition to this, the advanced education that is acquired in healthcare data analytics further sharpens your analytical ability through the development of critical thinking skills, which makes the holder of this degree more effective in solving problems in today’s complex healthcare organizations.
The master’s program commonly expands the curriculum from the undergraduate training and provides more specialized courses and applications.
Core Curriculum Components
The curriculum of a Healthcare Data Analytics degree is designed to provide a comprehensive understanding of both the technical and healthcare-specific aspects of data analysis. The coursework often includes:
- Data Acquisition and Management: Learn how to effectively source, clean, and prepare healthcare data, ensuring its accuracy, completeness, and readiness for sophisticated analytics, which are critical components of Healthcare Data Analytics.
- Statistical Analysis and Predictive Modeling: Obtain more proficiency in Statistical Analysis and Machine Learning Techniques to uncover patterns in Healthcare datasets that will help in the forecasting of trends and improving the potentials of the Healthcare Data Analytics.
- Healthcare Information Systems: Examine the technical structures behind gathering, saving and analyzing health records with emphasis on integration for use throughout any medical institution as well as other data optimization methods within them, which are essential components in Healthcare Data Analytics.
- Data Visualization and Reporting: Develop advanced skills in transforming complex healthcare data into clear, actionable visualizations and reports, empowering stakeholders to make informed decisions based on insights derived from Healthcare Data Analytics.
All these courses are strongly relevant in preparing you for Healthcare Data Analytics, and their importance cannot be underemphasized. The focus is directed not only on analyzing data but also on the analysis of what can be predicted, how it is better to work, and how to help the patient.
Career Opportunities and Responsibilities
Getting a degree in healthcare data analytics will equip you with the necessary expertise to perform important duties within the field. Key responsibilities in this field may include:
- Collecting and Organizing Comprehensive Healthcare Data: Ensuring that all relevant data is accurately gathered and structured for analysis, which is fundamental for effective Healthcare Data Analytics.
- Identifying Trends and Patterns Within Complex Datasets: This is what Healthcare Data Analytics should be doing – using algorithms to unveil useful information that can guide healthcare strategies and interventions
- Developing Predictive Models for Future Healthcare Scenarios: The power of Healthcare Data Analytics can be demonstrated through predictive modeling by applying statistical methods or artificial intelligence (AI) such as machine learning which helps predict trends, outcomes, and plan ahead for better healthcare services.
- Implementing Automated Processes for Efficient Report Generation: To make sure that reports reach decision-makers on time while also being accurate; this will help improve efficiency within the operational areas of healthcare data analytics.
Being essential in any healthcare organization, these duties are at the core of healthcare data analytics. Consequently, you will directly be involved with enhancing patient results, streamlining healthcare efficiency and strengthening the entire healthcare system.
Growing Demand and Career Outlook
The prospect of working in the healthcare industry is bright, but it is even brighter at the meeting point with technology. With the expected expansion of job opportunities in the healthcare sector, it can be seen that there are many prospects for people who want to work at this healthcare data analytics junction.
Since medical establishments keep using information-based findings more often than before, they will need more employees holding a degree in healthcare data analytics, hence raising their request for such professionals thus creating chances for promotions and career growth.
Why Is The Trending of Patient Data Important?
Here you will know the reason ‘why is the trending of patient data important.’. The fact is patient data analysis is very important in enhancing healthcare with the help of healthcare data analytics. Large-scale record reviews from many patients can help identify trends and correlations, which significantly enhances patient care quality and operational efficiency.
This data-driven approach (healthcare data analytics) enables the creation of tailored medicine that addresses the specific requirements of each patient when receiving treatment. Health practitioners are able to raise the standard of living, eliminate wastage of health resources, and reduce expenditure through the application of such trended information within their reach.
Personalized Medicine and Patient-Specific Treatments
One of the key benefits of trending patient data in Healthcare Data Analytics is the ability to implement personalized medicine. By examining detailed patient information, medical professionals can identify the right treatment for every individual.
Healthcare data analytics is focused on individuals and uses their medical background to suggest what could work best for them; this means that it not only increases success rates but also decreases the chances of negative outcomes or unnecessary interventions.
- Identification of Optimal Treatments: Patient data tracking can be used to find the most efficient treatments for each patient, which improves care quality and outcomes.
- Reduction of Healthcare Resource Waste: Personalized medicine eliminates unnecessary procedures or interventions hence healthcare industry can use its resources more efficiently lowering costs generally.
Advanced Analytical Techniques in Healthcare
Analyzing large amounts of health records using complex statistical methods may help us understand medical knowledge better and treatment outcomes. Healthcare data analytics aim at identifying clinical methods that work best with an intention of improving efficiency within healthcare systems.
- Designing Ideal Clinical Pathways: Recognizing changing trends from patient information with healthcare data analytics can assist with creating clinical maps that enhance patient care and operational efficiencies within health sectors.
- Data-Driven Decision Making: Healthcare data analytics enables the healthcare decision-makers to make right decisions thus achieving healthier patients besides resources optimization.
The Power of Big Data Analytics in Healthcare
The power of big data analytics in Healthcare Data Analytics stretches beyond bettering patient care alone as it helps reduce medical expenses, improve disease detection and prediction, and streamline the whole process of healthcare delivery.
Medical facilities may use big data that is available to them so as to create guidelines which will prevent rehospitalization from happening again, and enhance staff and equipment optimization while at the same time improving drug supply chain management.
- Lowering Healthcare Costs: It makes healthcare cheaper without compromising with its quality by locating areas that can be cut down on costs.
- Improving Disease Diagnosis and Prediction: Healthcare data analytics can make the disease diagnosis accurate through large datasets analysis thereby predicting future health trends which leads to early intervention and better patient outcomes.
Forecasting and Resource Optimization
Predictive analytics in healthcare data analytics is essential for predicting medical demands and efficiently allocating resources. Medical care providers can predict outbreaks, pinpoint patients at high risk, and develop individualized treatment models by tracking patient information. Such a novel method guarantees an appropriate distribution of resources which results into better general health service outcomes.
- Forecasting Hospital Bed and Operating Room Needs: Predictive analytics is useful for estimating the number of hospital beds required as well as when they will be needed.
- Optimizing Staff and Equipment: By trending data, healthcare providers can optimize the allocation of staff and equipment, reducing waste and improving operational efficiency.
Enhancing Drug Supply Chain Management
Improving drug supply chain management is a key use of trending patient data in healthcare data analytics. Healthcare institutions would be able to predict the demand for the drugs if appropriate trends in the patient data are evaluated. Thus, by ensuring that patients receive the appropriate prescriptions on time, healthcare data analytics assist avoid medication shortages.
- Improving Drug Supply Chain Management: Trending patient data can enable healthcare organizations to forecast drugs accurately thus ensuring continuous availability of required medicine thereby minimizing the chances of having less.
- Enhancing Patient Care: Healthcare data analytics can enhance patient care, reduce treatment delays, and improve overall health outcomes by making sure that medications are available.
Leveraging Predictive Analytics for Better Outcomes
Predictive analytics in healthcare data analytics allows medical professionals to identify high-risk patients, predict possible illnesses, and provide customized treatment programs that improve patient outcomes. Resource allocation is optimized by this new technique which is also responsible for better healthcare delivery as a whole.
- Identifying High-Risk Patients: Predictive analytics enables early intervention for those individuals at risk of developing certain diseases thereby preventing complications and improving outcomes.
- Creating Personalized Care Plans: Doctors are able to come up with personalized care plans based on their analysis of patients’ information leading to better health results and efficiency in delivering services.
Key Components of Healthcare Data Analytics
Converting large amounts of information into actionable insights that improve patient care and operational efficiency is what Healthcare data analytics does.
The essential elements of an effective healthcare data analytics system must be identified in order to properly utilize this power. These components work together to enable healthcare organizations to make decisions based on data, leading to improved patient outcomes and streamlined service delivery.
Data Collection and Integration
The foundation for any strong healthcare data analytics rests with robust data collection and integration processes.It is crucial that your analytical platform establishes direct connections with many data sources.
These sources may electronic health records (EHRs), laboratory findings, and even wearable devices used by patients. This is essential since it ensures that all aspects of patients’ wellbeing are taken into consideration during analysis thereby making it more accurate and holistic.
- Connecting to EHRs and Other Sources: A key component of Healthcare Data Analytics is the ability to seamlessly connect to Electronic Health Records (EHRs) and other data sources, ensuring that all relevant patient data is captured and integrated into the analytics platform.
- Comprehensive View of Patient Health: The use of various information sources in healthcare data analysis gives a complete comprehension of patient health necessary for correct diagnosis, planning treatment, and continuous care coordination.
Data Preprocessing
In Healthcare Data Analytics, data preprocessing is an essential step that must be completed before any meaningful analysis can begin. This phase involves determining the worthiness of information, addressing missing and abnormal values as well as standardizing formats among other steps. It ensures that the dataset is organized, consistent, and ready for accurate interpretation which is vital in making valid predictions or discovering useful insights.
- Evaluating the Quality of Data: In healthcare data analytics, it is necessary to work with good quality data. This means therefore correcting and confirming the data so that no issues that may distort the accuracy of the results arise.
- Standardizing Data Formats: Data preprocessing also includes standardizing data formats across various sources, which is essential for integrating and analyzing data from different systems in a unified manner.
Predictive Modeling
Predictive modeling is one of the most powerful tools within healthcare data analytics. Predictive modeling can be used to predict the pattern of the disease, risk assessment, and make appropriate recommendations for the treatment process. This tool is used in healthcare data analytics systems by applying machine learning and deep learning.
With the help of healthcare data analytics’ historical data extraction, these models predict future results so that healthcare providers can get involved earlier and design patient-specific therapies which ultimately improve outcomes.
- Forecasting Disease Progression: Using of predictive modeling in Healthcare Data Analytics gives the possibility for healthcare providers to know how diseases will progress in the patient and, therefore, allow them to alter the course of the disease on the positive side.
- Identifying High-Risk Patients: In healthcare information management systems, one of the most important uses for predictive modeling is identifying those people who are likely candidates for particular ailments or conditions so as to come up with targeted strategies for prevention and treatment. Through using healthcare data analytics for healthcare organizations can identify high-risk patients more effectively.
Data Visualization
Healthcare data analytics is made up of data presentation that converts complex data into actionable, easy-to-understand insights.These can be employed by care providers policymakers and executives among others on situations they would otherwise require technical proficiency in statistics or coding.
The use of charts, interactive dashboards and customizable reports in data visualization allows users to quickly identify key findings. Good healthcare data analytics must ensure all these observations are shared with everyone involved in the decision-making process so as to drive towards better results thus improving healthcare delivery at large.
- Interactive Dashboards: In Healthcare Data Analytics, it is vital to use dashboards whereby users can hover and manipulate data to gain more insights into specific data.
- Customizable Presentations: Customizable presentations in Healthcare Data Analytics enable healthcare professionals to tailor the display of data to specific audiences, ensuring that the most relevant information is communicated effectively.
Leveraging Key Components for Better Outcomes
The effective and enhanced realization and optimization of the major parts contribute to the improvement of the efficacy of Healthcare Data Analytics in the provision of healthcare services.
The necessary data must be obtained and incorporated into the models, and proper data preprocessing proves the data quality of the outcomes derived. Predictive modeling also assists medical professionals in preventing conditions that might occur in the future and data visualization makes it easier to present information that is understood by everyone.
- Improving Diagnoses and Treatment Plans: With the help of healthcare data analytics, doctors can make better diagnoses and create personalized treatment plans that work based on predictions. For example, doctors are using data to study how genes affect diseases. By understanding your unique genetic makeup, they can tailor treatments just for you. It’s like having a personal treatment plan based on your body’s blueprint.
- Enhancing Financial Efficiency and Resource Allocation: In addition, healthcare data analytics enables establishment to obtain maximum financial value. It also facilitates resource utilization which in turn result in better patient outcomes as well as sustainable health care system.Hospitals spend a lot on medicines, equipment, and staff. Using data, we can find ways to be smarter with money. For example, if we see that a particular medicine works better than another, we can buy more of the effective one and save money
Understanding Healthcare Data Analytics
Healthcare data analytics is an emerging tool in the modern healthcare sector. It helps professionals in healthcare sector in identifying the patterns that are helpful for improving various aspects of healthcare delivery by using both quantitative and qualitative These include choosing, gathering, processing, arranging and sharing information which is valid, dependable and current.
Knowledge gained from healthcare data analytics allows staff members to discover areas where they can work better, engage patients more efficiently and achieve better results through their services while increasing quality too.
Definition and Scope
In essence, Healthcare Data Analytics refers to the methodological examination of facts so as to come up with relevant insights capable of driving the decision-making process within medical facilities.
It includes data gathering, organizing and interpreting it for further actions being taken upon them. The range covered by this term is quite broad since it touches on various elements involved in healthcare such as efficiency or effectiveness, among others, even up-to-clinical outcome measures.
- Quantitative Data Analysis: The analysis of quantitative data encompass the systematic study of figures with the intention of eliciting patterns, trends and relationships. In healthcare it embodies information including; health records, patient’s data on their results, and the amount spent on health care services. This being the case, the following is a breakdown of some of the chief numbers that every healthcare provider should consider: For example, calculating the average days that pneumonia patient spends in the hospital can give direction to treatment.
- Qualitative Data Analysis: In contrast to quantitative analysis, qualitative data analysis focuses on understanding the context and meaning behind non-numerical information. This includes patient feedback, doctor’s notes, and other textual materials. This includes patient feedback, doctor’s notes, and any other form of textual content. Engaging with these qualitative particulars, healthcare providers can learn about patients’ experience, discover drivers of practice, and more generally reflect on health care. For instance, qualitative data gathering with patients on chronic pain treatment may be used to discover holes in a patient’s care plan.
Healthcare data analytics can help providers better understand their facility and the people who come to them for care. It allows them to decide what to do next with more information at hand, thus leading to better patient treatment quality, prudent financial use, and cost savings.
Evolution of Analytics in Healthcare
The Evolution of healthcare data analytics has been associated with technological developments in data management. Significant change in healthcare data analytics involves move from paper-based records to electronic health records. EHRs have completely changed how healthcare organizations access and analyze information by providing a single digital storage space for all patient details that can easily be shared across different medical service providers.
- Transition to Electronic Health Records (EHRs): The shift from paper records to EHRs has not only improved the accuracy and accessibility of patient data but also enabled the integration of data from various sources. This integration is vital in producing the big-picture analysis of any patient’s record since various healthcare providers can get a complete view of the patient in one place.
- Other Data Sources: In addition to electronic health records, other sources of healthcare information are critical for healthcare data analytics. They consist of computerized physician order entry (CPOE) systems’ clinical data, insurance claims, research data and the results from wearable medical devices among others. All these add to the pool of data which can be utilized for analysis on healthcare trends and enhancement of patient outcomes.
Impact of Analytics on Healthcare
The application of healthcare data analytics is not limited to enhancing patient experience and reducing cost. There are other areas where it is currently being applied as well:
- Epidemiology: Data analysis is very crucial for monitoring epidemics, comprehending the way contagious illnesses spread and finding ways to prevent them. Public health authorities depend on data from various sources thus enabling them to act on experiences that seek to enhance the health of people. For example, if the analysis revealed that the number of cases in a specific territory increased, then ventilators and health care employees were promptly shuttled in to that region, which proved to have a beneficial outcome.
- Clinical Trials: In clinical research, healthcare data analytics monitors patient outcomes; assesses the safety and effectiveness of new treatments; and ensures ethical & efficient conduct of trials among others. This approach supported by facts speeds up development processes for novel therapies bringing them into the market within short durations. For example a researcher would use prior data or data from a real trial to identify the correct sample size, the time for the trial, and the endpoints. This makes it easier to get the results that are required and also helps to minimize on the chances of unsuccessful trials which are often very expensive and time consuming.
- Genomics: One of the ways in which application of genomics in healthcare records is Health care data analytics to enhance Individualized medication. It becomes easy for scientists the notice the genetic traits associated with specific diseases and then proceed to provide treatment to patients based on their respective genetic make-up. For example, in cancer management, healthcare big data analytics allows physicians to learn about the gene characteristics of the affected tissue and which of them can be treated with particular medications. This approach has been quite effective in case of such types of cancer like lung cancer or melanoma where the treatments are selected depending on the changes in the genes, which trigger the formation of a tumor.
- Social Determinants of Health: Healthcare Data Analytics also takes into account social determinants of health like socio-economic status (SES), education level or environment where someone lives etc., which may affect patients’ outcome after receiving medical care. Providers should analyze these factors so as to design interventions that tackle root causes leading to disparities in health.
Key Stakeholders
Healthcare data analytics is successful through a combination of many actors with different roles within the healthcare system. Each stakeholder group encourages the use of healthcare data analytics by demonstrating that evidence-based findings lead to improved patient care operational effectiveness and long-term viability.
- Patients: Patients are central to efforts aimed at improving healthcare services because they are the direct beneficiaries of such improvements.Healthcare data analytics enables tailoring treatments according to individual needs thus enhancing patient outcomes and overall experience.
- Service Providers: Doctors, nurses, administrators and other healthcare providers use healthcare data analytics to improve how they deliver care. They can decide better on treatment plans by looking into patients’ data; manage patient care more effectively as well as identify areas for improvement.
- Decision-Makers: Healthcare executives together with policy makers depend on this approach when making decisions concerning various aspects about health like eligibility, finance and resources allocation among others so that they do not compromise quality while optimizing delivery systems towards sustainability based on their capacities.
- Payers: Healthcare financing and policy-making depend on insurance companies as well as public health organizations. Such stakeholders gain knowledge about cost-related tendencies within medical services, utilization rates among patients, and outcomes experienced by these individuals; thus enabling them to come up with strategies for pricing various policies so that they can encourage affordability while still maintaining quality standards based on healthcare data analytics.
The Rise of Healthcare Data Analytics
Healthcare Data Analytics is becoming very important in healthcare. Patient information in healthcare organizations is being handled in bulk.Because of this, healthcare analytics is now an essential tool for managing, evaluating, and utilizing this data to its fullest potential. In addition to that the technologies used here include artificial intelligence (AI) and machine learning (ML).
With the help of Healthcare data analytics healthcare organizations to make decisions that enhance patient care, streamline operations as well as save on costs. Indeed, according to the global healthcare data market projection, which was estimated at billions by 2023, no wonder it’s said that if you want to know how valuable something is. As long as the needs of healthcare organizations continue changing so will its functions change too.
In addition to that healthcare data analytics improves patient safety and enhances healthcare standards. Analyzing different types of trends or patterns contained within datasets, thus helping with early warning signs identification around health risks, among other things related to treatment hence enabling personalized provision by healthcare organizations.
This, in turn, leads to better outcomes for patients’ well-being post-treatment, even including mental satisfaction levels achieved during such processes! The field itself expects an increase rate of about 19% per annum up until 2035, meaning not only more jobs but also shaping future healthcare systems through the utilization of big dataset knowledge.
Benefits of Data Analytics in Healthcare
Healthcare Data Analytics is an innovation that is revolutionizing healthcare. This technology comes with a number of advantages, some of which include; enhanced patient handling, efficiency, and reduced cost of handling patient’s care. The following are some key advantages:
1. Improved Patient Care and Outcomes
Healthcare data analytics improves patient care and outcomes. Providers can develop personalized treatment plans for each patient based on analysis of large amounts of information about their specific needs which is more likely to work best for them.
For example, if a patient’s oxygen levels start to drop unexpectedly, the analytics system can immediately alert the medical staff, even before the patient shows visible symptoms. This allows doctors to intervene earlier, perhaps administering oxygen or adjusting medication, potentially preventing a serious complication.
- Personalized Treatment Plans: Data analytics for healthcare organizations can customize treatments based on various factors using analytic tools hence increasing chances of recovery among patients.
- Early Detection and Prevention: It helps healthcare organizations to ensure that it identifies any health issues or future health issues before they become major problems. Hence, they can deal with such matters before those become critical problems.
- Increased Patient Involvement: Healthcare data analytics can also put patients in a position to know more about their own health and, in this way, participate in their treatment. When patients are active, they are more likely to adhere to the given treatments and hence enhance their health standards.
2. Enhanced Operational Efficiency
Healthcare Data Analytics enhance patient care and the smooth running of healthcare organizations. Through examining data about patients within the system, healthcare providers can identify ways to work more efficiently.
For instance, they might discover that a particular time of day consistently sees a surge in patient visits, leading to long waits. By using these insights, the hospital can adjust staff schedules, ensuring more doctors and nurses are available during peak hours. They can also streamline discharge processes to free up beds more quickly, allowing for faster patient turnover.
- Better Resource Use: Through data analytics, healthcare institutions can ensure that their resources, including staff and equipment, are used optimally. For instance, healthcare organizations may forecast how many individuals will require attention on a given day and ensure adequate workforce provision to meet this demand.
- Shorter Wait Times: Delays can be identified and resolved by medical service providers through analyzing information regarding patients’ data through the system. As a result, waiting periods for patients would decrease thereby enabling them to receive treatment quicker.
- Simplified Administration: In addition, healthcare data analytics has the capacity to automate repetitive tasks as well as enhance data management processes which lightens the administrative burden on health workers thus giving them more time for taking care of patients.
3. Better Disease Tracking and Prevention
Healthcare data analytics is very important when it comes to preventing and tracking diseases. Healthcare organizations can recognize the patterns of diseases spreading by looking at information from electronic health records, wearable technology, and public health databases. It helps them take precautions against outbreaks even before they occur.
This method worked extremely well during the COVID-19 pandemic as healthcare data analytics were utilized to track how the virus spreads and inform decisions on public health.
In chronic disease management like diabetes or asthma, care data analytics helps in discovering trends within patient data which may require changes in treatment plans for better control over these conditions. By using healthcare data analytics, it becomes possible for public healthcare providers to identify specific areas where there are more cases of illnesses. It allows them to plan interventions that target those regions while increasing prevention.
For instance, the Centers for Disease Control and Prevention (CDC) in the United States used healthcare data analytics to monitor COVID-19 trends in real-time. By analyzing data from hospitals, testing sites, and even social media, the CDC could identify patterns of outbreaks, predict surges in cases, and allocate resources like ventilators and vaccines more effectively.
4. More Accurate Diagnoses
Healthcare data analytics improve diagnosis accuracy since it provides the healthcare provider with a comprehensive view of the data that may otherwise be unrelated. Through the use of lab results, patient notes, and other databases and records, healthcare data analytics enables a diagnosis to be made more accurately and at the right time thus minimizing on misdiagnosis and assuring that patients receive the right treatment.
Also, predictive analytics which is a subset of healthcare data analytics enables the providers to predict the likely occurrence of health complications. Through the use of genetics data and comparing patient details with large databases of medical records, healthcare data analytics assists healthcare organizations in identifying patients who are at risk and thus prevent them from being misdiagnosed hence improving the patient’s health.
Let’s say this hospital uses an advanced analytics system that integrates patient records, lab results, and even genetic information. The system can compare the patient’s data with millions of other records to identify patterns and correlations that might not be immediately obvious to a human doctor. For example, it might notice that patients with similar symptoms, combined with a particular genetic marker, were often diagnosed with a specific, rare condition.
5. Reduced Healthcare Costs
Healthcare data analytics has been proven useful in improving the efficiency and effectiveness of utilization of resources in healthcare delivery thus lowering the costs. This means that through the application of healthcare data analytics, organizations are able to enhance their performance and decrease their expenditure hence, consequently spending less on the delivery of quality services.
Also, healthcare data analytics is useful in enabling providers to offer care at a lower cost by identifying the tests and treatments that are not required and thus using the resources only when they are required. This not only cuts on the costs but also prevents the occurrence of adverse effects from procedures that may have been avoidable.
Also, through the analysis of costs as well as the overall billing processes, healthcare data analytics enhances financial management which is evident in cutting down costs and generating more revenue for the healthcare facilities.
Challenges in Implementing Healthcare Analytics
Patient care and healthcare management can be changed by the use of Healthcare Data Analytics. Its advantages include the enhancement of patient satisfaction, the enhancement of operational performance, and the reduction of expenditure. However, there are still some challenges that need to be addressed in order to reach this potential.
Below are listed what we consider as main challenges when implementing Healthcare Data Analytics:
1. Data Security and Privacy Concerns
Some of the biggest issues that Healthcare data analytics encounter are the issues of security and privacy of sensitive patient information. Due to the vast amounts of personal information, including medical and genetic information being collected and stored, this information is valuable but can also be easily stolen or leaked.
Since any violation of the patient’s privacy results in adverse effects like identity theft, financial loss, and negative impact on the healthcare organization, it is imperative to safeguard the patient’s information. Hence, healthcare data analytics must embrace strong security technologies such as encryption, firewalls, and access control.
Also, healthcare data analytics is governed by the rules of patient data privacy as seen in HIPAA of the United States of America among other countries. The consequences may include legal sanctions, fines, and, above all, the loss of the patient’s confidence. In addition, an important problem is the question of how to provide data access while maintaining privacy.
Although it is impossible to treat patients without collecting all the necessary information about them, the information must be protected. Thus, the measures which can be taken to ensure that only authorized personnel have access to sensitive information include strict access controls.
2. Integration of Diverse Data Sources
For the purpose of healthcare data analytics it is often necessary to merge different kinds of information together. Some examples can include electronic health records, wearable devices, lab results, insurance claims and social determinants of health. These various sources may have different formats, standards or even technologies which makes this process very complicated and difficult.
One significant challenge in doing so is what we call ‘data silos’. This happens when pieces of information are kept separate in systems that cannot share it resulting in fragmented and incomplete data. Removing these barriers is crucial to ensure that healthcare data analytics functions effectively.
Another obstacle faced by healthcare data analytics is the interoperability problem since different health systems use incompatible software as well platforms. Achieving interoperability will require standardizing formats for the storage or representation of data across all platforms while ensuring communication flows smoothly between them too.
Additionally, structured data like dates should be included along with unstructured data such as doctors’ notes and images where necessary. These are some of the ways that are helpful for the integration and analysis of diverse data types, which are vital for obtaining more precise and comprehensive views in healthcare data analytics.
3. Data Quality and Standardization Issues
Information must be of high quality and standardized for healthcare data analytics to work. Poor data quality can result in incorrect findings and this may affect the care given to patients negatively as well as decision-making processes. In order to ensure that healthcare data analytics is based on accurate and consistent information, there should be cleaning up of records systems.
Another issue is standardization where different providers use various terminologies thus making it difficult for them to share data or conduct joint studies because they have different formats for presenting their findings. This can be addressed by adopting universal sets like SNOMED CT or ICD which are commonly used in the industry today.
It is also important that we ensure the completeness of our datasets; if we have incomplete records then there will be gaps in knowledge that could lead to wrong conclusions being drawn during the analysis stage. Thorough collection methods such as establishing comprehensive protocols should therefore be put into place so that all necessary details may be captured thereby improving efficiency in healthcare data analytics.
4. Regulatory Compliance
The healthcare industry faces significant challenges with regulatory compliance when implementing Healthcare Data Analytics. Strict regulations such as HIPAA in the U.S. and GDPR in Europe impose complex requirements on data collection, storage, and sharing. Non-compliance leads to severe fines therefore careful interpretation is required while dealing with these regulations.
To make sure of obedience, you have to keep data confidential, put access controls in place and use information ethically. Healthcare providers should match their practices with the changes in rules governing healthcare data analytics. These policies are essential in safeguarding patients’ privacy rights but they can also impede innovation. Organizations need to balance regulatory adherence with adopting new technologies to enhance their Healthcare Data Analytics capabilities.
5. Resistance to Change Within Organizations
The main reasons behind resistance towards the implementation of healthcare data analytics are the already present corporate culture and doubtfulness about new technologies.
To surmount this challenge, it is necessary that we have some efficient tactics for managing changes which may involve making communication transparent, giving all-round training and providing strong support systems. Work staff will require time and other resources in order to adjust to advanced tools and workflows related to healthcare data analytics.
The involvement of leadership is important if success has to be achieved during the implementation process. Securing buy-in from organizational leaders helps drive the adoption of Healthcare Data Analytics and ensures the allocation of necessary resources.
By addressing cultural resistance and providing enough training, healthcare facilities can facilitate the smooth transition towards new technology, thus maximizing benefits associated with such systems like HCA (Health Care Analysis).
Overcoming the Challenges
Healthcare Data Analytics is an area with a lot of potential, but it also has some challenges that must be overcome by healthcare organizations. Robust cybersecurity investments, privacy law compliance and data interoperability enhancement through standardized formats and integrated systems are among the necessary steps toward this direction.
Moreover, for Healthcare Data Analytics to work effectively there should be governance frameworks which ensure that data quality is not compromised; in addition, audits need to be carried out regularly so that cleanse processes can take place where necessary.
In order for establishments to fully utilize Healthcare Data Analytics they should work hand in hand with legal teams who will help them comply with rules and regulations stipulated by relevant authorities while keeping their knowledge up-to-date on any changes taking place within this field.
It is through fostering cultures supporting innovation coupled with change management strategies supported by good leadership skills including continuous learning opportunities among staff members were resistance towards adopting such new technologies can be addressed successfully within healthcare organizations.
Types of Healthcare Data Analytics
Healthcare data analytics is a vast field that includes various ways of extracting useful knowledge from massive amounts of healthcare information. Different types of healthcare data analytics help medical professionals make decisions, improve patient outcomes and manage organizations better. You need to understand different classifications of healthcare data analytics if you want to use data effectively in the health industry.
1. Descriptive Analytics
Descriptive analytics serves as the basis for all other methods used in healthcare data analytics. It involves examining of the historical records to understand past or current decisions made on health matters. This type of healthcare data analytics is centered on transforming the raw data into meaningful form so as to provide an easier method to analyses the outcomes, quality & efficiency of the healthcare systems.
- Understanding Past Trends: This type of healthcare data analytics enable medics to know about patterns over time depending on patients’ information like admission rates into hospitals among others such as physician performance or resource utilization rates. Looking at what has worked best before through healthcare data analytics, organizations find out areas they need to improve upon thereby learning from their own experiences.
- Reporting and Benchmarking: Descriptive analytics in healthcare data analytic is often used to generate reports that benchmark healthcare performance against industry standards. For example, healthcare organizations can use healthcare data analytics to compare their patient outcomes with national averages, helping them identify areas where they excel or need improvement.
- Quality Improvement: Healthcare organizations are also use healthcare data analytics to discover patterns pertained to the patient’s improvement and healthcare quality with the help of past information. This type of information can be deployed healthcare data analytics to promote quality improvement interventions in the provision of better healthcare to patients as well as the improvement of organizational efficiency.
2. Diagnostic Analytics
While diagnostic analytics is not explicitly mentioned in the provided information, it plays a crucial role in Healthcare Data Analytics. A closer look at data to understand why things happened, diagnostic analytics is important in healthcare data analytics for uncovering the reasons for a health problem or an operational bottleneck.
- Identifying Root Causes: Diagnostic analytics within healthcare data analytics enables healthcare organizations to investigate the causes behind particular healthcare results. For instance, if there is an unexpected increase in patient readmissions, healthcare data analytics can help identify the factors contributing to this trend, such as inadequate follow-up care or medication non-compliance.
- Operational Efficiency: In addition to clinical applications, diagnostic analytics can also be used to analyze operational data. For instance, healthcare organizations can employ healthcare data analytics to know why some sections are not meeting expectations while others may use it to determine what is causing longer patient waiting hours.
- Supporting Clinical Decision-Making: What diagnostic analytics does is that it enlightens caregivers about why there are some problems in the industry and this helps them make informed decisions regarding their own practice which will eventually lead to improved patient outcomes.
3. Predictive Analytics
Predictive analytics is one of the most effective tools in Healthcare Data Analytics. It looks at past events to predict what will happen later on, thus letting healthcare givers get ready for risks, identify invisible patterns and plan for the days ahead.
- Forecasting Patient Outcomes: Predictive analytics can help healthcare providers forecast how patients will respond to different treatments or medications. Predictive models within healthcare data analytics is able to recommend which treatments may work best for a particular patient by studying information from similar cases in the past.
- Risk Anticipation: Expectant analytics is very useful in spotting patients who are most likely to develop particular diseases. For instance, healthcare data analytics can use patient records to forecast who will contract diabetes or heart disease. With the help of healthcare data analytics, healthcare organizations can prevent these risks and hence improve patient outcomes.
- Planning for Epidemics: Healthcare data analytics is essential in planning for epidemics. In times of public health emergencies like pandemics, healthcare data analytics provide predictive analytics that could be employed in projecting how fast an ailment may spread while assisting in proper distribution of resources. If health providers can forecast the possible location and timing of an outbreak, they will be better positioned to deal with it.
- Improving Diagnoses: Another use of healthcare data analytics lies in improving how accurate diagnoses are. To do this, what they do is look into patterns in patient data, which can enable physicians to identify possible health issues earlier on so that their diagnoses become faster and more exact.
4. Prescriptive Analytics
Prescriptive analytics is the most advanced form of Healthcare Data Analytics. It advises particular actions on the basis of intricate models that bring together a variety of information sources, thus this type of healthcare data analytics allows medical institutions to make better decisions in different situations.
- Recommending Patient Services: Healthcare data analytics through prescriptive analytics can suggest the best services or treatments for patients based on their unique medical history and current health status. For example, it can recommend which therapy is likely to yield the best outcomes for a patient with chronic pain.
- Optimizing Healthcare Operations: In addition to clinical applications, healthcare data analytics can be used to optimize healthcare operations through prescriptive analytics. it can find out the best methods of helping underperforming departments like recommending for staff changes or upgrading equipment.
- Reducing Risks: In healthcare data analytics, one of the major advantages of prescriptive analytics is risk mitigation. Prescriptive analytics identifies the most appropriate actions to take in complicated medical scenarios thus enabling healthcare providers make decisions which minimize risks while enhancing results.
Data-Driven Strategies for Healthcare Cost Reduction
To maximize operational efficiency and enhance the quality of care, healthcare data analytics is a cost-effective approach that can be employed. Healthcare organizations digitize their records to eliminate paper-based systems, they are able to make better decisions using real-time information through healthcare data analytics.
Efficiency in the operating room, correct staffing levels, and preventing unnecessary readmissions, among other benefits, can be achieved through analysis of this data, which are all cost-saving. Additionally, optimizing the supply chain through healthcare data analytics reduces errors and improves inventory management, further driving cost savings.
Key Applications of Data Analytics in Healthcare
The healthcare industry has been significantly transformed by healthcare data analytics that provides tools for informed decision-making among medical professionals. All these decisions are not only beneficial, especially in the provision of patient care, but more importantly, they contribute to the overall organizational management of healthcare organizations.
The incorporation of large data sets from multiple sources into the healthcare sector has sparked new opportunities for improving healthcare and personalizing patient care. Here’s a deeper look at the key applications of Healthcare Data Analytics:
Leveraging Electronic Health Records (EHRs) and Electronic Medical Records (EMRs)
Healthcare data analytics is a foundation of using electronic health records (EHRs) and electronic medical records (EMRs), which contain all-encompassing patient data such as drugs, symptoms, lab findings and treatment outcomes. Medical providers can get to know the overall health journey of their patients and risk factors by use of this information.
EHRs/EMRs give a comprehensive overview of a person’s medical record thus helping them track how conditions develop as well as response to various treatments and potential future dangers if any. This kind continuity is important in understanding patient wellness over time.
Accessing detailed patient histories through healthcare data analytics leads to better decision-making ability. For example; doctors may analyze patterns in past diagnoses coupled with current test results so that they can come up with correct assessments about illnesses or suggest appropriate interventions.
Predictive Analytics for Personalized Treatment Plans
Another aspect of healthcare data analytics is prediction which entails utilizing machine learning for assessing data in the past and present to make decisions in the future. For healthcare organizations, this is an invaluable tool that enables patient risk identification and development of personalized treatment plans.
Such factors as medical history, present conditions and lifestyle choices are analyzed by predictive analytics to reveal patients at high risk for adverse events like readmissions or complications. This allows early intervention from caregivers who offer preventive care while tailoring treatments to decrease such risks.
Moreover, healthcare data analytics makes personalized medicine possible by examining a person’s genetic makeup, clinical records plus their way of life. Predictive models can help determine how a specific patient would react to some medication thus reducing trial-and-error processes during prescribing drugs leading to more accurate prescriptions hence improving patients’ wellbeing.
Integration and Streamlining with ERP Systems
Enterprise Resource Planning (ERP) systems are critical in managing healthcare institutions whereby Healthcare Data Analytics plays a crucial role. Integration of various data using ERP systems within operations creates efficiencies, eliminates gaps in documentation and supports business intelligence.
For healthcare organizations consolidation of clinical, administrative and financial data helps provide accurate information that is updated regularly and required for informed decision-making as well as improved patient care.
Additionally access to all relevant information gained by healthcare data analytics to practitioners improves continuity of service delivery thereby reducing errors resulting from fragmented care while promoting collaboration among providers involved in disease management which translates into coherent care pathways across multiple settings.
Personalized Medicine and Proactive Prevention Strategies
The role of Healthcare Data Analytics in personalized medicine is to study an individual’s genetic makeup, lifestyle choices, and surroundings. This enables care givers come up with treatment plans that are unique for every patient thus increasing efficiency of treatment as well as promoting proactive prevention strategies.
Healthcare providers can now fit treatments depending on genetic profiles and lifestyle factors through healthcare data analytics which reduces adverse effects while increasing health outcomes. This ensures that patients receive only the best treatments available thereby lowering risks involved with them.
Apart from treatment, thanks to healthcare data analytics it has become possible to practice personalized medicine. Health workers are now able to know patients who are at higher risk of getting diseases such as heart problems or cancer so that they can take preventive measures before symptoms appear like regular screening exercises or lifestyle modifications.
On the whole, healthcare data analytics makes treatment more individualised hence better results are obtained since invasive procedures will be minimized leading to shorter recovery periods and improved quality of life among patients too.
Improving Clinical Decision-Making with Analytics
Healthcare Data Analytics is a game changer when it comes to clinical decision-making; this means that medical professionals have more information at their disposal which leads to better decisions being made within shorter time frames.
For instance, real-time healthcare data analytics, evidence-based treatment recommendations, and error-reduction tools can be used by health practitioners to offer quality services and save lives while reducing unnecessarily incurred costs. Here is the detail of how Healthcare Data Analytics is enhancing clinical decision-making:
Real-Time Analytics at the Point of Care
Healthcare Data Analytics has significance where the analytics can be performed in real time at the patient care touch points. They allow the healthcare providers to access the patient information in real time with all the details including the medical history, diagnosis, and treatment information with the final results.
It also helps to make decisions faster and more effectively since they do not have to dig through irrelevant information to get to the most important details.
By getting data from various sources, real-time healthcare data analytics paints a comprehensive picture of a patient’s health which helps doctors make quick informed decisions regarding their treatment. It brings about better efficiency not only in the treatments offered to the patients but also in relation to the costs incurred in the right or early treatments.
Evidence-Based Treatment Recommendations
Evidence-based medicine (EBM) combines scientific research findings with clinical expertise and patients’ preferences when making decisions about individualized care. Healthcare Data Analytics enhances Evidence-Based Treatment by facilitating the identification, appraisal and application of high-quality evidence in clinical practice settings.
Healthcare data analytics informs clinically meaningful questions by employing big data techniques to identify trends in patient data to develop questions that guide the improvement of the healthcare delivery systems with the intention of enhancing the quality of life as well as the health of people who depend on these systems.
Healthcare data analytics also speed up the identification of the most appropriate evidence through rapid scanning of medical literature as well as clinical trials thus ensuring that decisions are based on current studies.
Once evidence has been gathered together Healthcare Data Analytics helps in appraising its validity critically while considering whether it applies broadly enough or just few cases were used during its development process before being implemented into practice. Providers can then apply this evidence to patient care, using healthcare data analytics to monitor outcomes and adjust treatments as needed.
When integrated into EBM, healthcare providers will be able to make better decisions about what works best for whom under different circumstances; leading to improved patient outcomes and care tailored towards individual needs.
Reducing Medical Errors
To prevent medical mistakes, healthcare data analytics plays an important role in providing real-time monitoring tools, alerts, and risk identification. It can proactively identify high-risk patients through the analysis of electronic health records (EHRs) and other sources of data thereby leading to timely interventions for preventing complications.
Healthcare data analytics systems that monitor patients’ data in real-time are able to pick out any anomalies such as vital signs or lab results among others and immediately send notifications to doctors which reduces errors greatly. Moreover, healthcare data analytics also helps with preventing medication-related errors by looking into prescription information where potential drug interactions or dosage problems could be detected.
Additionally, healthcare data analytics ensures clarity in communication between different medical practitioners while at the same time improving accuracy as well as completeness in recording events thus enhancing safety for all patients involved.
Patient-Centric Analytics
Healthcare data analytics are changing the way care is given. This enables more personalized, proactive care; greater patient involvement; and better healthcare by using the best techniques including artificial intelligence (AI), machine learning (ML) and natural language processing (NLP). Healthcare data analytics leads to the adoption of value-based healthcare models that rely on remote monitoring, among other things.
Patient Engagement and Satisfaction
Optimize patient experience management by collecting and analyzing data across all touchpoints along a person’s healthcare journey. Structured feedback surveys, as well as clinical outcome measures, constitute some examples of structured data sources while call center transcripts or online reviews make up some instances of unstructured information sources.
Advanced healthcare data analytics such as machine learning (ML) and natural language processing (NLP) can be used to identify patterns or predict needs from such huge amounts of diverse records, thereby facilitating very proactive personalized service delivery.
Remote Patient Monitoring
In this type of care provision model, patients’ physiological measurements are taken from home or any other place outside hospital settings over time so that their condition can be monitored closely even when they are not nearby.
Modern RPM systems rely on connected medical devices that automatically send readings back to doctors’ offices through wireless networks. Initially, it was used mainly for cardiac conditions but now covers many other diseases like hypertension and diabetes mellitus.
Population Health Management
Make informed strategic decisions about interventions targeting groups at greatest risk based on predictive modeling using big datasets sourced during routine operations like electronic health record systems deployment, among others, thus saving lives through proper use of knowledge acquired once processed within population healthcare data analytics frameworks that support comparative effectiveness research in healthcare while also ensuring cost containment throughout these processes until completion without sacrificing quality standards at any stage.
Improving Patient Care through Data-Driven Insights
Data-based healthcare is expected to greatly transform the patient experience. You will be able to comprehend what your patients require from you and how best you can deliver it when you collect information from different sources, such as patient feedback, medical records or even through live monitoring.
To enable data-driven patient experience, you can:
- Collect patient feedback through surveys, focus groups, and social media
- Leverage patient data to personalize experiences using healthcare data analytics
- Implement patient portals for access to health information
- Use predictive analytics through healthcare data analytics to anticipate patient needs
- Offer telemedicine services for remote care
By aggregating and analyzing claims data, prescription data, EHR data, and reporting data through healthcare data analytics, you can create a comprehensive patient health record.
This all-encompassing look promotes transparency in care, continuity and better clinical quality results. Real-time access to longitudinally linkable data allows authorized providers to make informed decisions at the point of care which may promote disease prevention and raise satisfaction rates among patients.
Big Data Technologies in Healthcare
Data Integration and Interoperability
The control of data in healthcare was completely changed by big data technologies. Different databases can be used to process structured and unstructured data on a very large scale.
Through the healthcare data analytics system, International Classification of Diseases (ICD) coding helps combine patient discharge records with electronic death certificates as well as medical claim data. The main aim for such integration is that it enables easy sharing of information between different healthcare providers leading to better delivery of care services.
Cloud Computing and Storage
Healthcare data storage and accessibility were made easier through cloud computing. Now, it is possible to store huge amounts of clinical or molecular information at affordable prices. Professionals within healthcare can now access patients’ details from wherever they are located, which improves the flexibility of delivering services.
Cloud-based systems also offer the much-needed infrastructure for telemedicine hence enabling video consultations, among others ,while ensuring security during data sharing.
Machine Learning and AI
Healthcare data analytics has been enhanced by machine learning coupled with artificial intelligence, which is now the driving force behind most innovations in this field. Predictive analysis may be done using AI where patterns are detected from big datasets so as to predict outbreaks of diseases or patient deterioration.
AI-powered diagnostic tools have high accuracy rates when it comes to interpreting medical images, thus making it easier for doctors to detect conditions such as cancer or cardiovascular diseases. Machine learning algorithms can also go through genetic information thereby allowing personalized medicine approaches that recommend treatments based on individual patients’ needs.
Healthcare Data Analytics: Opportunities and Challenges
Improvement in patient care as well as operational efficiency is one of the many benefits of healthcare data analytics. The prediction of diseases can be enhanced through big data which may also help in personalizing treatments and optimizing allocation of resources. Large-scale analysis of patient data through healthcare data analytics can show patterns which enable doctors to make better choices.
On the other hand, there are challenges associated with establishing these systems. Security concerns about privacy for data are high on the agenda; hence strict measures must be put in place to secure patients’ sensitive information. Furthermore, integrating different sources of data while ensuring its quality can prove difficult too.
We have to address those challenges if we want healthcare data analytics to be used for more positive results. In your journey through this field, balancing innovation against ethical considerations will be necessary in transforming service delivery and results within healthcare systems
Virtelligence The Best to Integrate Electronic Health Records with Analytics Platforms
In today’s rapidly evolving healthcare environment, the successful integration of Electronic Health Records (EHR) and analytics platforms is essential for optimizing patient care and operational efficiency. Healthcare organizations that need to make their processes more efficient will find no better partner than Virtelligence. This is because when it comes to healthcare technology integration, the Virtelligence has unmatched experience.
Why Choose Virtelligence?
Virtelligence is a leader in healthcare technology consulting and has successfully supported the organizations to implement and optimize Electronic health record systems. Starting from the beginning with planning and strategy development up to complete implementation and optimization; Virtelligence brings experienced resources required for seamless integration of EHRs into healthcare facilities.
Virtelligence is famous for offering affordable, flexible service packages aiming at delivering good outcomes without spending too much money. They can be trusted by any healthcare provider who wants to improve its technological setup because they are able to adjust themselves wherever necessary during any given project thereby becoming reliable partners in this area.
Expertise in Leading Platforms
Virtelligence’s expertise extends across some of the most widely used healthcare platforms, including:
- Epic: Virtelligence guarantees that your EHR system is fully optimized to enhance patient care and simplify operations. With knowledge of many Epic installations, their consultants can manage upgrades, implementations as well as optimizations that are complicated to make sure that they work seamlessly in your healthcare setup.
- Cerner: Virtelligence is good at integrating Cerner systems so they assist providers in utilizing the platform for effective clinical workflows and better management of information. From initial deployment through ongoing maintenance their staff is capable of handling any task thereby ensuring maximum efficiency with which Cerner operates.
- Workday: Apart from Workday being an HR system, in the healthcare industry it has been used as such by many facilities. This therefore means that Virtelligence has wide-ranging experience with this Workday, particularly when it comes to fitting together different healthcare systems already in place within an organization; their competence enables enterprises to maximize benefits brought about by using Workday, which leads to improved financial operations relating to HR functions within such establishments.
Comprehensive Services
Virtelligence offers a wide range of services that tackle all points of electronic health records (EHR) and analytics integration. Their comprehensive approach ensures that all elements of your healthcare technology ecosystem are working together effectively:
- Implementation and Optimization: Virtelligence guides organizations through the entire process of implementing new EHR systems or optimizing existing ones. Virtelligence specializes in making certain that all EHR systems are integrated with other platforms and achieves interoperability, thereby enabling smooth transfer of data between departments and facilities.
- Integration and Interoperability: Sharing data effectively among different systems is vital in modern healthcare. Virtelligence specializes in making sure your EHR system is integrated with other platforms completely achieving interoperability, thereby enabling smooth exchange of information between departments and facilities.
- Advisory Services: Virtelligence provides expert advice on EHR strategy, helping organizations make informed decisions about technology investments and system enhancements. Their advisers collaborate closely with your staff members in creating plans that match with your objectives while enhancing the overall performance of the whole system itself.
- Project Management: Complex EHR projects require careful coordination and management. Virtelligence provides project management services which ensure that integration or optimization projects remain within budget confines, stays on schedule and achieve all necessary milestones.
- Custom Application Development: When off-the-shelf solutions don’t meet your needs, Virtelligence can develop custom applications that integrate seamlessly with your existing EHR system. This helps ensure that clinical workflows are supported by appropriate technologies while aligning them towards organizational goals for operational efficiencies.
- Staffing and Hiring: Getting the right staff is key to successful EHR and analytics integration. Virtelligence provides staffing & recruitment services which connect you with skilled professionals who will drive forward your technological projects.
- Global Talent Solutions: Beyond local markets, Virtelligence reaches out worldwide with talent solutions so that you can access the best resources wherever they may be situated. This approach at the global level guarantees that your institution benefits from up-to-date skills as well as new developments in healthcare technology.
Transforming Healthcare Systems
Virtelligence is more than just a consulting firm—they are a partner in transforming healthcare systems. They provide the full range of services, including EHR and EMR integration, ERP solutions, project management and staffing which helps healthcare organizations navigate through the complexities of technology integration so that it works best for them. The main aim of this company is not just to make a healthcare facility work but to make sure that it runs at its highest level of efficiency possible.
When you are introducing a new EHR system, upgrading an existing one, or even looking to strengthen your overall technology strategy, Virtelligence has all the skill sets and resources required to achieve these objectives. Such things enable health providers to concentrate on their core business which is offering quality care to patients while at the same time ensuring that such activities are supported by an appropriate underlying technological platform.
Revolutionizing Patient Care The Role of Healthcare Data Analytics
Revolutionizing patient care the role of healthcare data analytics has become increasingly crucial in enhancing treatment outcomes and improving overall healthcare efficiency. Healthcare data analytics has transformed patient care by examining vast amounts of medical data to uncover meaningful insights. Sophisticated analytics raises the quality of patient care, the overall organization of care delivery, and the efficiency of the services provided.
Another advantage of healthcare data analytics is that it enables one to forecast outcomes and, therefore, aggressively prepare for the patient’s needs. With this, it becomes possible for one to identify potential health hazards as well as come up with individualized treatment plans. Prescriptive analysis goes a step further by recommending what should be done in order to achieve desired ends while taking care that it is relevant to each patient’s needs.
Moreover, healthcare data analytics is also essential in promoting health equity and finding areas where workflow efficiencies can be made better. When one has an all-round comprehension about their clients which takes into account social determinants of health; they are able to get more profound insights that will guide them towards effective targeted outreach programs. This approach driven by facts is changing how medical services are delivered thereby fostering prevention and enhancing patient experiences in general.
Ethical Considerations in Healthcare Analytics
In healthcare data analytics, it is important to address ethical concerns. Among these are data privacy and security. Data leakage becomes more likely as the volume of information being processed increases. Additionally, algorithmic bias can perpetuate societal prejudices and lead to misdiagnosis among underrepresented groups.
Ways of mitigating this include ensuring that datasets are diverse and implementing algorithms that reduce bias. Trust can only be built with transparency and explainability. Making decision-making processes transparent through models such as explainable AI will enable you to appreciate their strengths as well as shortcomings easily enough.
For this reason, research methods should be shared openly alongside codes used during experiments so that global researchers can have a chance to assess how an algorithm performs across different populations. Privacy, accuracy, and security should be included in algorithm design if they are to work effectively.
As an AI user, it’s your duty as a healthcare giver or practitioner; therefore, make sure that you ask questions about the functionalities of algorithms from developers so that you may know what they can do or not due to biasing factors associated with them.
Transforming Clinical Decision-Making
Healthcare data analytics has revolutionized clinical decisions by enabling more personalized care provision based on evidence of what works best for each patient thus leading to better outcomes while reducing costs through this process. Evidence involves making use of advanced technologies backed up with strong proof according to research findings thus making informed decisions that will generate positive results.
Evidence-Based Medicine
EBM integrates clinical experience; and patient values together with the best available current scientific knowledge when coming up with treatment options. Systematic approaches under EBM help select the most effective interventions rooted in good foundation research, hence greatly improving the healthcare provided by practitioners EBM has several key features, including:
- Integration of clinical expertise and patient preferences
- Use of the most reliable and up-to-date evidence
- Consideration of individual patient characteristics
- Continuous evaluation of treatment outcomes
Clinical Decision Support Systems
Clinical Decision Support Systems (CDSS) have emerged as powerful tools to augment your complex decision-making processes. These systems integrate patient-specific information with evidence-based guidelines, research findings, and best practices to provide personalized treatment recommendations, dosage calculations, and potential drug interactions. CDSS can help you:
- Reduce medical errors
- Improve treatment outcomes
- Enhance clinical workflows
- Stay compliant with industry standards
Precision Medicine
Genomic advances have opened up the field of precision medicine, where treatment can be tailored based on an individual’s genetic makeup. You can identify disease risk, drug response or reaction to side effects by studying the DNA of a patient. This enables you to come up with treatment plans that are specific to each person thus increasing their effectiveness and reducing adverse reactions.
Enhancing Patient Care and Outcomes
Early intervention and personalized care have been made possible in healthcare by predictive analytics. With sophisticated healthcare data analytics tools for the prediction of health results as well as the identification of high-risk patients, caregivers are able to offer targeted support at an early stage before readmission occurs thereby improving outcomes for patients.
Predictive Analytics for Early Intervention
Predictive models can detect potential risks to health before they become severe by using large amounts of healthcare data. The aim is to intervene early enough so that individualized treatment plans can be developed, leading to better care outcomes.
Personalized Treatment Plans
A broad range AI-driven analytical tools collect information from different sources which creates a complete view about one’s health status, hence enabling physicians to design customized care strategies that will increase chances for successful treatment outcomes and satisfaction among patients through healthcare data analytics.
Remote Patient Monitoring
By utilizing Remote Patient Monitoring (RPM), practitioners are able to keep track of vital signs continuously, even when patients are at home. Real-time recording shows how a person is fairing on health-wise thus allowing faster decision making in regards to therapy while also cutting down unnecessary costs within healthcare delivery system.
Future Trends in Healthcare Data Analytics
As technology continues to evolve, the future of Healthcare Data Analytics looks increasingly promising, with several emerging trends poised to transform the healthcare industry:
- Artificial Intelligence and Machine Learning:Healthcare Data Analytics has been identified to be on the verge of significant evolution by the incorporation of AI and machine learning. These technologies will allow a healthcare provider to process even larger datasets more efficiently and make a more accurate and effective prediction of patient outcomes. This integration will also help to create individual approaches regarding cases of patients, as a result, the treatment outcomes will be optimized.
- Real-Time Analytics: Real-time Healthcare Data Analytics will become a crucial factor as well. It is due to this trend that healthcare providers can make an instantaneous decision that has been informed by technology and this can lead to a vast enhancement in the quality of patient care and also increase operational efficiency. Real-time analytics will enable physicians and other healthcare givers to respond effectively and in the moment to an altered state in the patient’s health hence improving the patient’s health conditions.
- Patient-Centered Analytics: Given that patients are taking an active role in the decision-making on their health, there will be a progression towards patient-centered Healthcare Data Analytics. This trend will focus on applying data for the purpose of improving patient satisfaction, thus making healthcare services more personalised. The culture of patient analytics for health care allows for improving the satisfaction of patients and, therefore, the results of treatments, helping physicians to become more patient-centered.
- Integration of Wearable Devices: Mobile health and wearable technologies is the other trend that characterizes Healthcare Data Analytics. These devices transmit constant information regarding the health conditions of the patients so that the problems can be detected early and attended to appropriately. As healthcare technology initiatives, these devices will require integration with the framework used by Healthcare Data Analytics to help better utilize the data from them as to also improve the ability to provide proactive personals care.
- Data Privacy and Security: Since Health Care Data Analytics have becoming a vital aspect in the Health Care System, patient data privacy and security is a major concern. With the increasing amount and connectivity of healthcare data, there will be growing focus on applying innovative security solutions. Healthcare Data Analytics has been designed in a way to protect the patient’s data and allow free flow of data for enhancing patient’s care.
Final Thoughts
It is very evident that healthcare data analytics plays an important role in healthcare decision-making also in patient care. Thanks to the integration of new-age technologies, the healthcare industry is now able to use evidence-based decisions for the betterment of their patients and the general quality of healthcare. AI plus machine learning today has paved the way for a lot of innovations in the field of medical today because of the possibility of analyzing huge data information.
In other words, healthcare data analytics is still an emerging field that has revolutionized patient care and will define the future of healthcare. As we have discussed, from predictive analytics to population health management, this domain includes promising prospects for professionals aiming for career growth. Based on research findings, healthcare has increasingly become data-driven and, therefore, needs innovation and education in this fast-growing area.
FAQs
What are the benefits of data-driven decision-making in healthcare?
What are the benefits of data-driven decision-making in healthcare? Data-based decision-making in healthcare comes with a lot of pluses. One major benefit is improved patient outcomes through individualized treatment plans. Secondly healthcare data analytics enhances operational efficiency by enabling better resource allocation.
Promoting preventive care as well as early detection of diseases are other ways through which this approach cuts down costs in general health service provision. Also, strategic planning with healthcare data analytics backed up by data supports informed decisions making among healthcare providers, while increased involvement of patients yields more fruitful care delivery.
Finally, but importantly, healthcare data analytics with research and innovation thrive well in such an environment, leading to advancements in medical treatments and technologies.
What is predictive analytics for healthcare decision-making?
Healthcare predictive analytics is the use of past data to estimate what is likely to happen in the future so as to better patient care and streamline operations. Healthcare providers can prepare for potential medical conditions, optimize treatment strategies, and allocate resources most appropriately by studying historical data trends or patterns.
This method, ‘Healthcare Data Analytics, ’ detects diseases early enough before they spread too widely, thus saving lives besides fostering good decision making, which in turn leads to improved patient results coupled with reduced costs, thereby making healthcare proactive towards addressing problems at their earliest stages hence offering efficiency while caring for individuals.
What is the data analysis process in healthcare?
A decision analysis model in the field of healthcare data analytics is defined as a tool for comparing various medical decisions based on such factors as cost, probability, and quality of life. It enables healthcare professionals to input a wide range of values, including the cost of treatment and the quality of the health-related quality of life of a patient, and exercises the probable impacts of a broad range of alternatives and choices.
Consequently, in these outcomes, healthcare agents can effectively and efficiently determine better solutions that could meet their bottom line and, at the same time, cater to the health needs of individuals. This is more so in the area of public health, where maximum impact has to be made with available resources without compromising on quality.
What is an example of medical decision making?
Medical decision-making involves evaluating patient information and healthcare data analytics to determine the best course of action. Let’s take an example of a medical decision with the help of healthcare data analytics.
A doctor may decide on drug therapy that requires intensive monitoring for potential toxicity, using healthcare data analytics to track patient responses and adjust treatment as needed. Another instance could be the decision to proceed with elective major surgery, carefully considering patient risks and data-driven insights to ensure the procedure’s success.
In emergencies, the decision to perform urgent surgery might be made swiftly, relying on real-time data and analytics to manage the situation effectively and ensure the best possible outcomes for the patient.
What is clinical data analytics?
Clinical data analytics means the capturing, processing and usage of clinical data for the enhancement of healthcare plus the organization’s productivity. Healthcare data analytics incorporates data from Electronic Health Records, clinical trial results, and patient outcomes in a bid to make a healthcare diagnosis, establish patterns, ensure efficiency in healthcare delivery and ensure medical safety compliance.
A clinical data analyst is a critical participant in this process as he/she is responsible for data accuracy and application of data in healthcare-related decisions within the organization.